How the Age of Intelligent IP Video emerged – and its implications on the future of privacy.
A Short History of Video Surveillance 1930s - Present Day
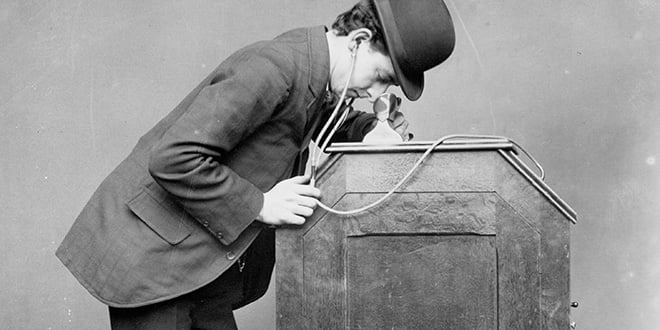
1880: The first movie cameras were developed by Thomas Edison and William Dickson. in 1883 they would put on a demonstration of the the first motion picture.
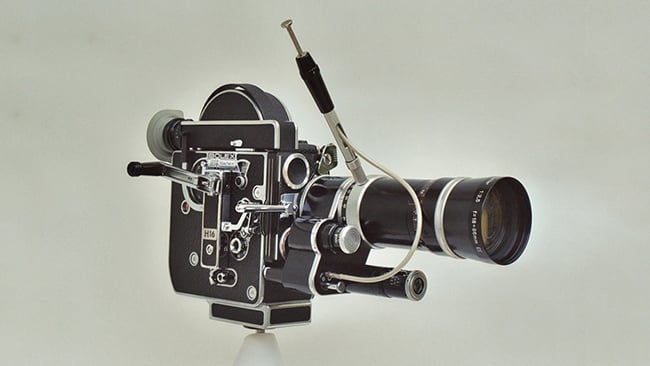
1939: Miniaturized portable cameras began to appear – just in time to be used in WWII for covert troop surveillance.
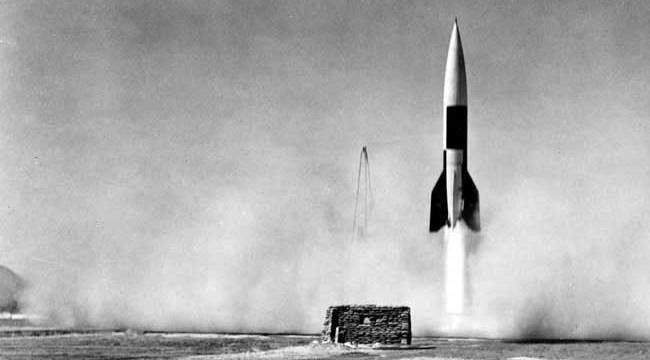
1942: Closed Circuit Television (CCTV) appeared in Germany and was used to capture the launch of V2 rockets. Very similar to the way SpaceX uses a VMS to capture their rocket tests.
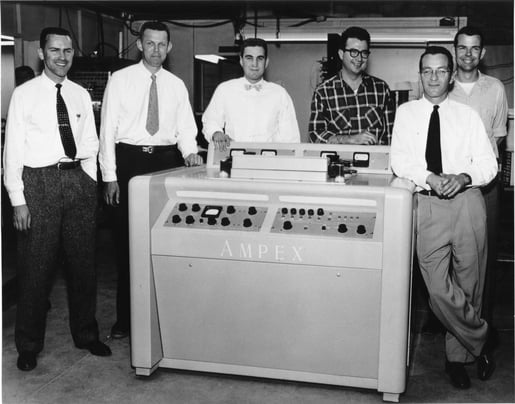
1951: The arrival of the first commercially viable VTR. Launched in 1956 by the Ampex Corporation, the VRX-1000 came with a $50,000 price tag (equivalent to ~$470,000 in 2019)
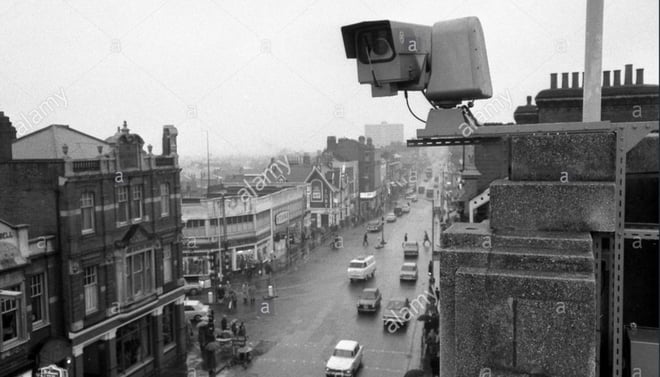
1960s: Police began to utilize CCTV to protect dignitaries and to investigate criminal behavior in public places.
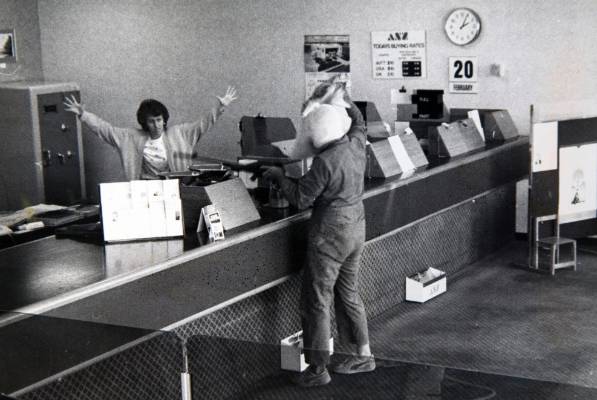
1970s: The VCR hits main stream and CCTV begins to be adopted outside the government, mostly by Banks and high value commercial retail users.
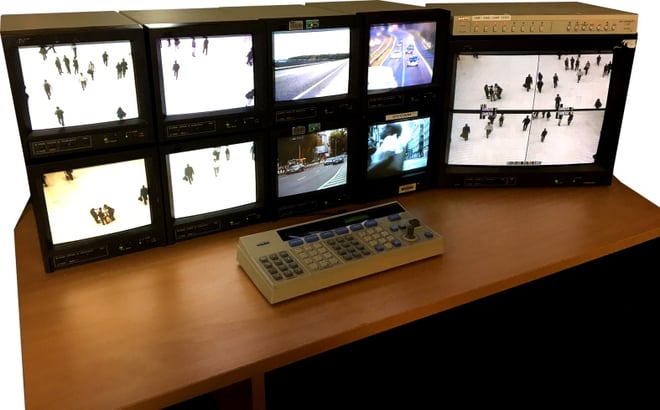
1980s: CCTV begins to be deployed across all market segments – including private homes – powered by more affordable cameras and more feature-rich VCRs.
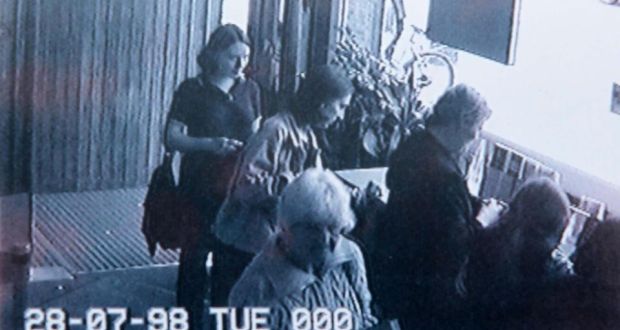
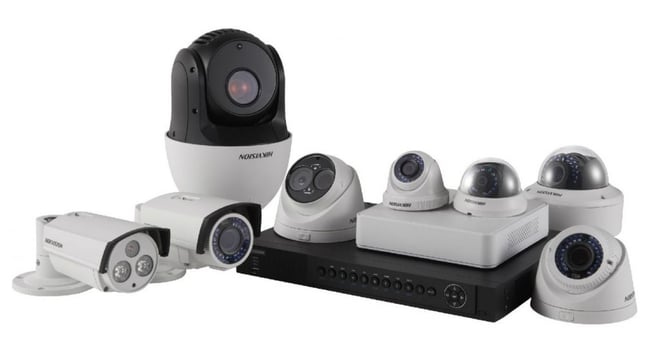
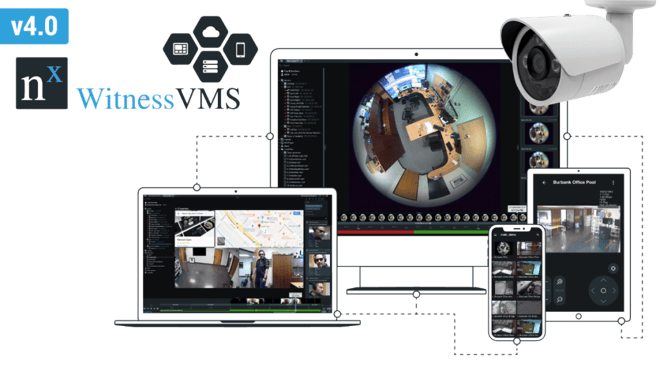
A new Millennium and the introduction of VCA to mainstream markets.
In the mid to late 2000’s companies like ObjectVideo began introducing video content analytics which used a combination of computing algorithms to analyze captured video in real time to look for and notify CCTV system operators when certain behaviors were identified. The premise of video analytics when launched was that it could potentially reduce costs and human error by using machines to identify events for operators. Whereas in the past a security guard would have to man the monitors 24/7/365 to catch would-be trespassers or criminals, video would now be able to identify and track certain events and behavior on camera and alert owners when these are detected.
Early VCA applications included tampering (modifying or blocking a camera’s view), motion analysis (directional motion), and even simple object recognition (like detecting faces or people). And while these initial algorithms performed fairly well in controlled laboratory environments they struggled to function properly in real world environments for several reasons.
First, detecting the presence of someone or something on an image requires analyzing many things including the difference with the previous frames (to detect movement), the direction of motion, the size of the object, and many other variables. Traditional detection models required extensive computing resources and could only be deployed in environments with tightly controlled lighting conditions. This meant they were expensive – both to buy and to maintain. As a result some companies sought to combine traditional sensors and detectors with cameras via I/O inputs, but their accuracy tended to be low as they detected all motion – regardless of the type. This resulted in much frustration in the industry as the promise of video content analytics exceeded the practical capabilities.
Additionally, as hardware and software improved VCA continued to use the same basic principles to identify behavior – it analyzed pixels based on changes that occurred, with limited effectiveness in determining who or what was in a frame (image) of video.
As such there was one solution left: teach the computer to recognize objects in video.
Deep Learning & IP Video Surveillance
Deep learning is a technology present in nearly every person’s life at this point in time. The photos and videos you capture with your smartphone are analyzed and used to train neural networks which, in turn, get ever better at recognizing objects and people.
The best way to do this, it turns out, is not to use human generated algorithms or throw computing power at the problem. It is to use real-world examples and attached structured metadata. By working with large tagged data sets companies could begin to “teach” neural networks to recognize objects and their behavior – and most importantly – could do so with a high level of accuracy.
By continuously training a deep learning neural network with new data and giving it the ability to train itself companies could finally solve the challenges of VCA in a more consistent, dependable manner. This allowed companies to use structured data sets from a wide variety of environments and different types of camera views to begin to overcome the environmental challenges involved to make VCA applications more accurate and reliable.
Deep learning has become such an effective approach that during the past decade is has become useful in nearly every type of application – text translations, autonomous driving applications, and even video content analysis. The power that can now be dedicated to such learning tools is gigantic, and the results are getting better every day. In video surveillance software, this has translated into better tools for detecting people, objects, movement, and behaviors.
Google’s Cloud Vision, Microsoft’s Computer Vision API, and Amazon’s Rekognition engine can be used by any company to add VCA capabilities into their product lines. There are also a myriad of additional software-as-a-service (Saas) providers like VisionLabs and Anyvision that provide dedicated A.I. powered VCA applications for specific use cases like face recognition or people detection.
Hardware vendors are also now moving towards making AI focused products. Hikvision, Dahua, Hanwa Techwin, and many other companies are now offering IP cameras with built-in neural networks capable of doing advanced VCA.
And then there are the chip manufacturers in a race to provided SoC platforms to computing device manufacturers. NVIDIA is one of the major players, pushing for deep learning tools in their enthusiast and professional grade graphics cards, giving them a large advance in the field. More players have been entering the field, and competition is fiercer than ever. What simply used to be specialized tools for high performance computing and numerical crunching has since become something that is now driving how hardware and even the infrastructure of servers, software and networks are made. As AI becomes more and more needed, the breadth of products they make has increased, leading to products such as Jetson – a small, deep learning focused chip that can be integrated into many devices.
Companies in the IP Video Management field like Network Optix are constantly innovating to make it easier for innovators to create A.I. enabled video management products with platforms like Nx Meta VMP.
A.I. Enabled Video Systems and Privacy

The democratization of IP video surveillance, the ubiquity of IP cameras, and the coming of the A.I. age brings huge potential benefits including capturing more real-world data and enabling Google-for-real-world applications which can increase the situational awareness and relevant safety of humanity on a massive scale.
But these trends in video technology can also be abused. While it is very convenient for any store owner or person just looking to keep an eye on their home or business, AI powered tech can become a major inconvenience for privacy-conscious people. The sheer number of IP cameras being deployed on a daily basis makes it very likely that anyone’s steps throughout the day could be very easily retraced, effectively ending privacy as humanity has known it for millennia.
Of course, video cameras are not fully to blame for this. The amount of metadata captured by smartphones and Internet connected software about a single person’s activities throughout the day is staggering, and can allow any bad actor to follow and influence individuals with targeted, tailored content that plays to their psychographic profile.
As such governments will need to step in with legislation that balances the privacy needs of society with the benefits of such technology. Laws such as Europe’s GDPR (General Data Protection Regulation) or California’s CCPA (California Consumer Privacy Act) restrict what personally identifiable information can and cannot be collected, kept and used and must be refined as technology progresses.
Other approaches that can be taken include technology which obfuscates the identity of people on the camera or VMS level, restricting access to personally identifiable information to those who have the proper authority and understand the gravity of the potential impacts of unnecessarily identifying individuals.
With every new leap in technology comes challenges, both technical and social. As we adapt our hardware and software to make the world a more transparent place we must not forget to consider the potential social impact. Here at Network Optix we will continue to strive to make a VMS that balances the usefulness of A.I. while considering the privacy implications for individuals – and we encourage all companies to take the same approach.